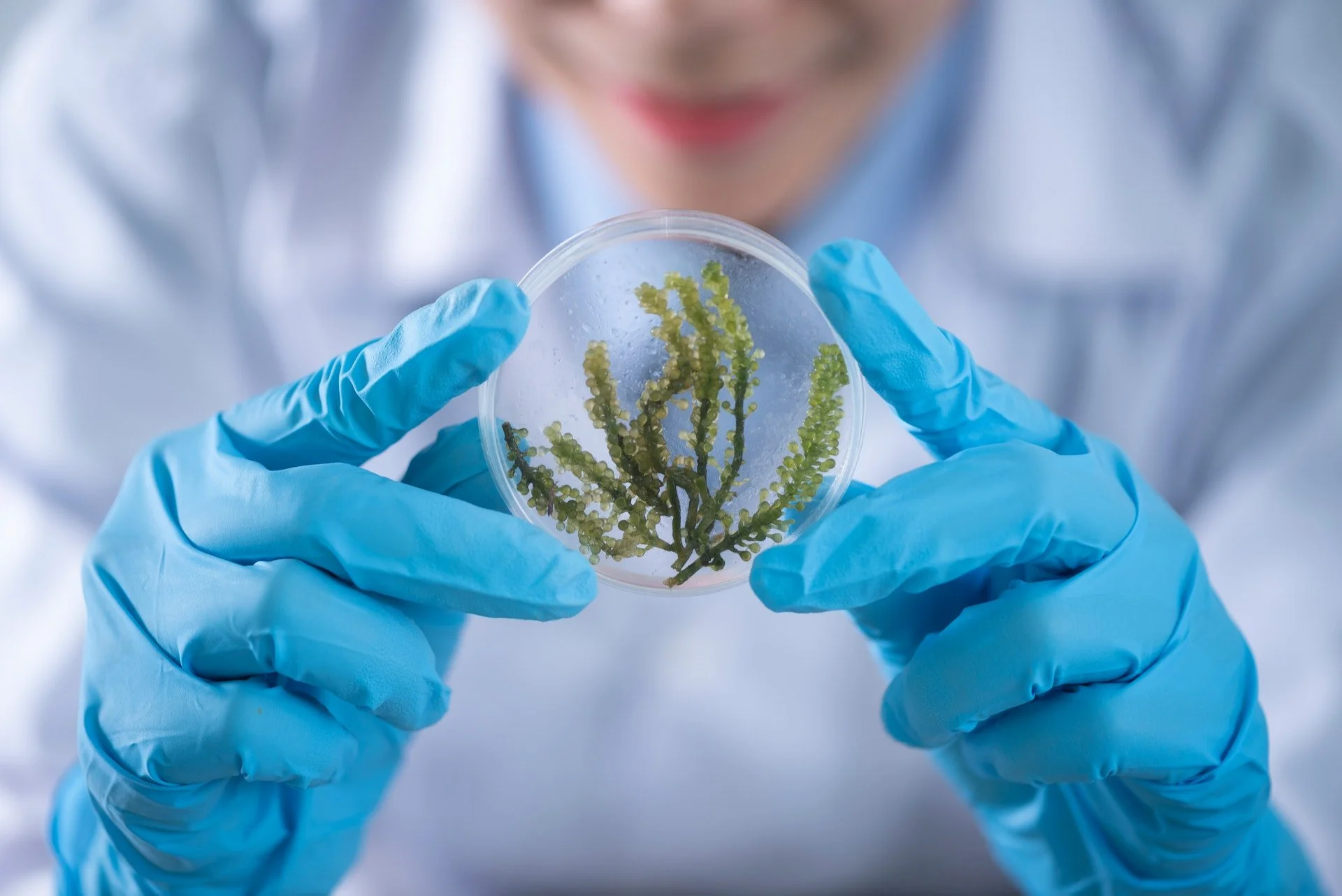
Environmental Science
Ground-breaking new methodologies
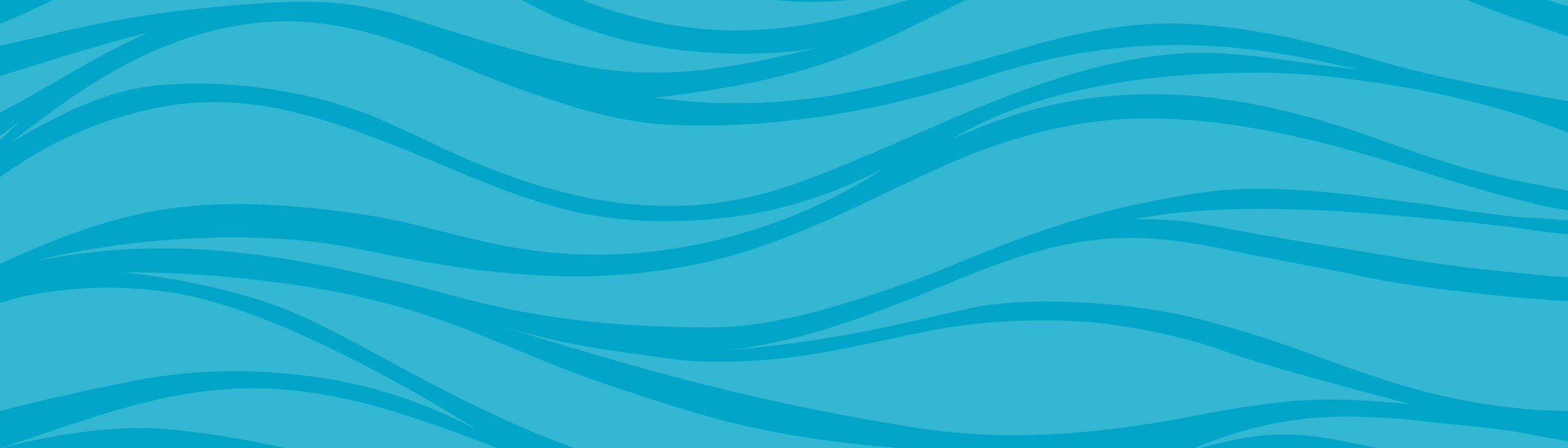
Environmental DNA for Biodiversity
DNA-based tools are used to assess biodiversity in habitats. Each sampling effort lets us look at nature through multiple lenses – from microbes to megafauna – enabling assessment of overall ecosystem status at the same time as detecting the presence of key species of economic or conservation importance, or those that pose a threat to the habitat. This ensures long-term ecosystem resilience while providing opportunities for participatory monitoring and engagement of local communities and stakeholders.
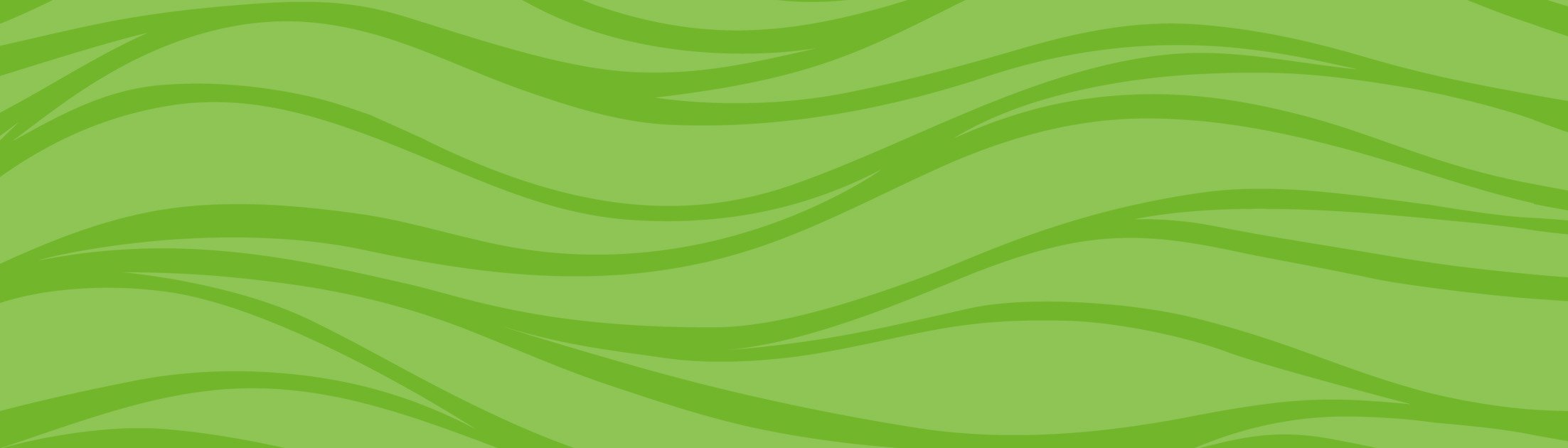
Environmental Metabolomics
Environmental metabolomics is used to characterise the interactions of differing organisms with their environment. This has many advantages for studying organism–environment interactions and for assessing organism function and health at the molecular level. This molecular level of understanding habitats then informs our understanding of the challenges, opportunities, permanence and threats we face, and how we can best address them
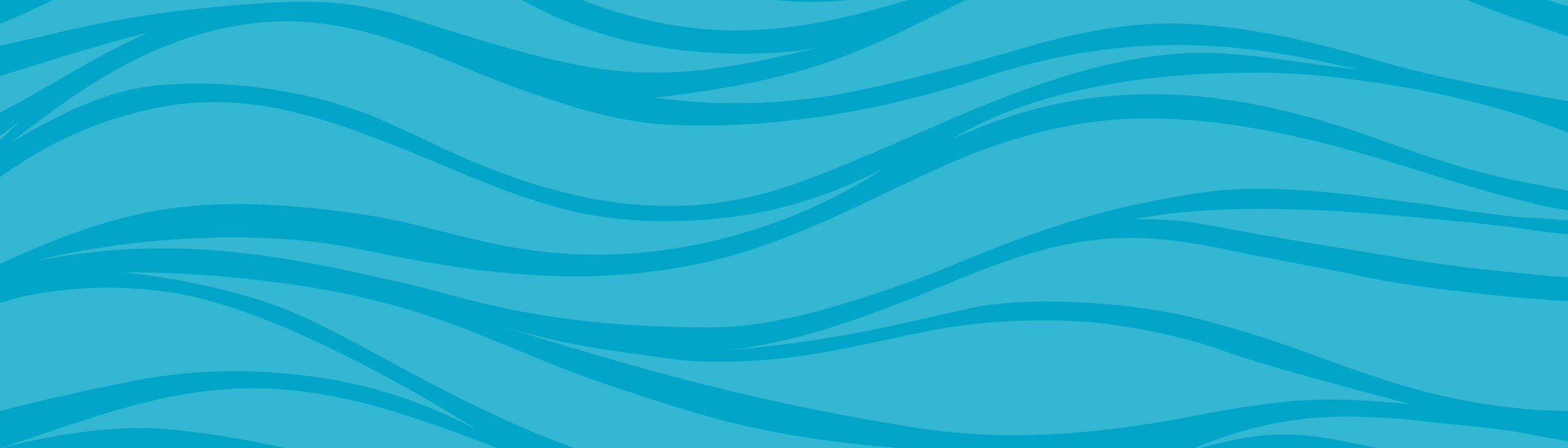
Genome Sequencing of the Microbiome
The field of microbiome research is a further evolution of microbial ecology which combines classic microbiology with new sequencing techniques, novel bioinformatics, biostatistics and math modeling. This permits a much deeper understanding of the microbial communities residing at the sites we work with, their interspecies relationships and the host-microbiome interaction, for microbiomes living either in or on animals and plants.
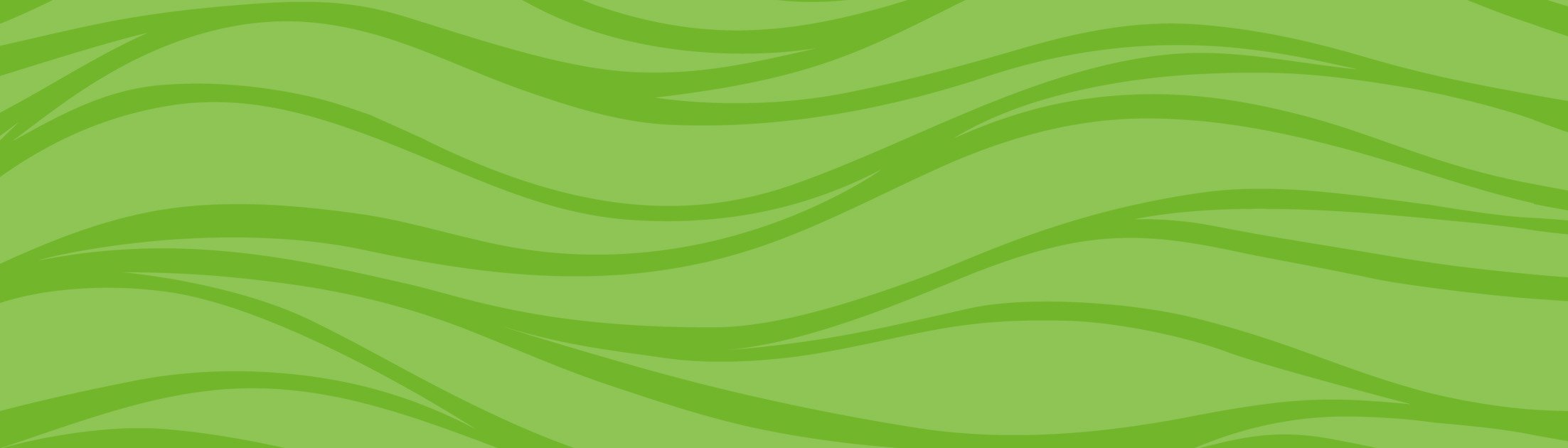
Measurement of Carbon Sequestration
Through photosynthesis, the rainforest turns Carbon and water into carbohydrates, or sugar, in order to grow. The trees then releases Oxygen back into our atmosphere. Biomass is the organic material of all living organisms and, in trees, about half of it is carbon. Our models allow us to accurately measure the amount of carbon (sequestration) in any area of rainforest - both above and below the ground - through a mix of field measurements and remote-sensing, establish a 'trend' (eDatum) and estimate future levels.
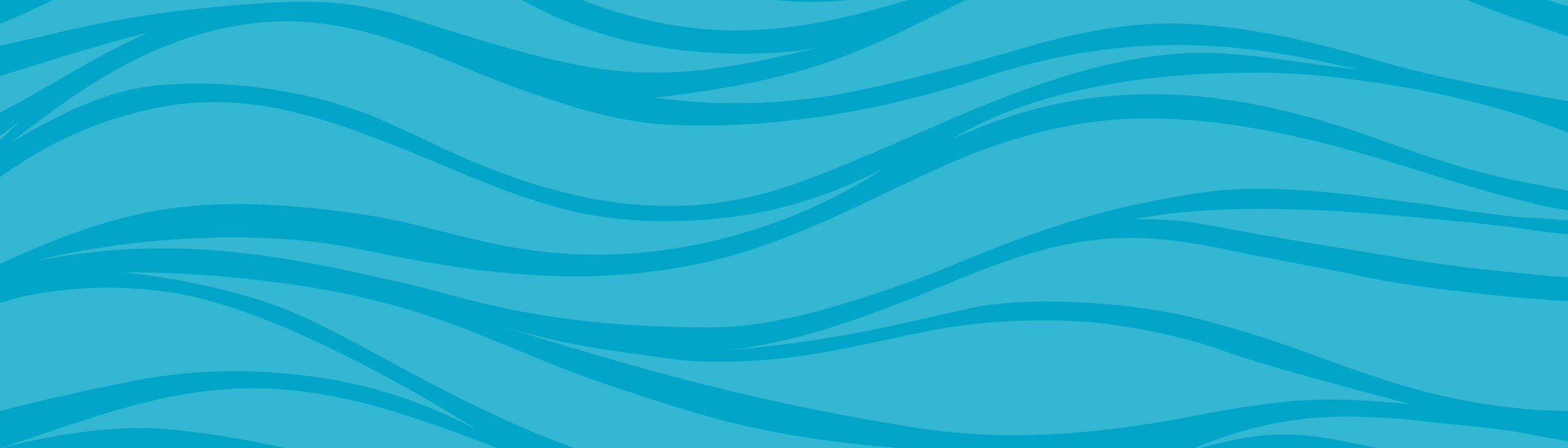
Machine-Learning
We take all of the data we've generated from both our earth observation work and our work 'in the field' and run it through our machine-learning algorithms, to deliver a framework to inform decision-making which is accessible, understandable, transparent and verifiable.